Demystifying the complexity of AI by ensuring Interpretability and Transparency
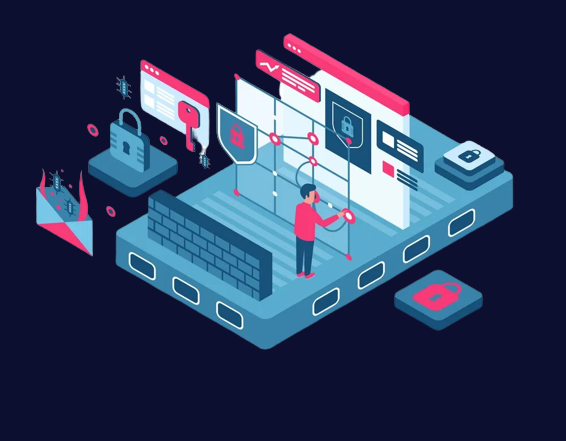
Gain insights into your model across granularities from row to model level
Employing informed data driven strategies leading to error minimization and enhanced decision making
Simulate input data elements to visualise the impact of change on the predicted outcome
Deploy transparent and trustworthy AI/ML Models with more confidence
Gain insights into your model across granularities from row to model level
Employing informed data driven strategies leading to error minimization and enhanced decision making
Simulate input data elements to visualise the impact of change on the predicted outcome
Deploy transparent and trustworthy AI/ML Models with more confidence
TuringXai uses Individual Conditional Expectation (ICE) charts to visualize how changes in one of the predefined top input parameters affect the model’s predictions while keeping all other input parameter constant. This provides a clear understanding of how the model is using each input parameter and how it is making its decisions